0
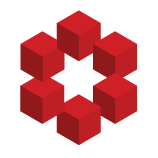
Let $X_n, X,Y_n,Y$ be real valued random variables. I know that if $X_N\stackrel{(d)}{\rightarrow}X$ and $X_N\stackrel{(d)}{\rightarrow}Y$ then $X$ and $Y$ have the same distribution. Now I asked myself does this imply that $X=Y$ almost surely?
I think that this do not work in general because ...